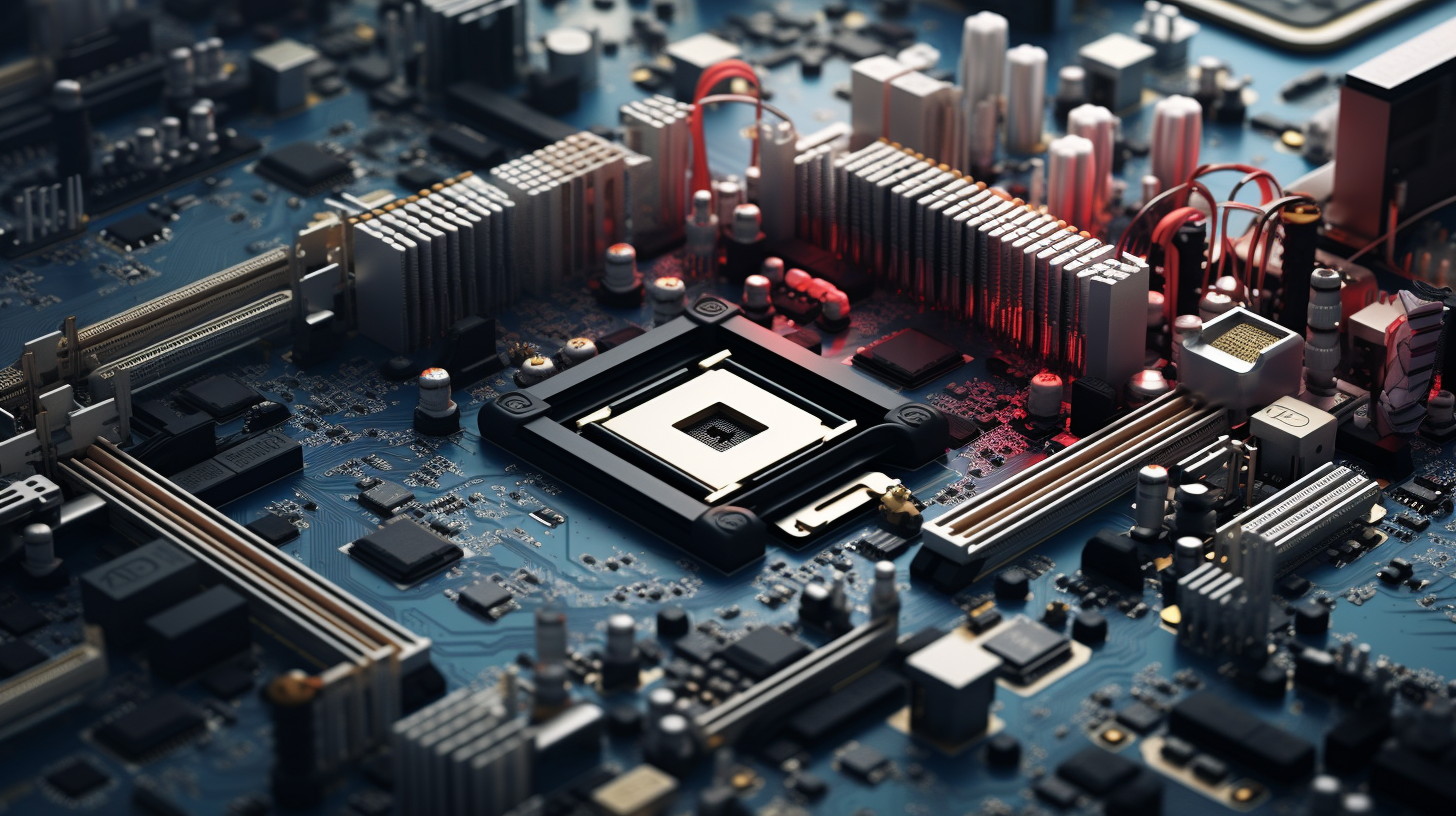
Swift and CoreML Advanced Techniques
In the sphere of machine learning, the ability to leverage pre-trained models can serve as a significant advantage, accelerating the development process and enhancing application performance. Swift, Apple’s powerful and intuitive programming language, provides seamless integration with Core ML, enabling developers to easily incorporate pre-trained models into their applications.
Core ML supports a wide variety of pre-trained models that can be readily used for tasks such as image classification, text analysis, and more. By using these models, developers can harness complex algorithms and vast datasets, which have already been fine-tuned, allowing them to focus on creating innovative features rather than training models from scratch.
To demonstrate how to leverage a pre-trained model in Swift, let’s think the use of a vision model for image classification. First, you need to include the model in your Xcode project. For instance, you can add a model like MobileNetV2, which is optimized for mobile devices and offers excellent performance for image recognition tasks.
After adding the model, you can implement it in your Swift code as follows:
import CoreML import Vision import UIKit class ImageClassifier { private var model: VNCoreMLModel init() { guard let mlModel = try? MobileNetV2(configuration: MLModelConfiguration()) else { fatalError("Failed to load the model") } model = try! VNCoreMLModel(for: mlModel.model) } func classifyImage(_ image: UIImage) { guard let ciImage = CIImage(image: image) else { fatalError("Failed to convert UIImage to CIImage") } let request = VNCoreMLRequest(model: model) { request, error in guard let results = request.results as? [VNClassificationObservation] else { fatalError("Unexpected results") } self.handleClassificationResults(results) } let handler = VNImageRequestHandler(ciImage: ciImage, options: [:]) do { try handler.perform([request]) } catch { print("Failed to perform classification: (error.localizedDescription)") } } private func handleClassificationResults(_ results: [VNClassificationObservation]) { if let firstResult = results.first { print("Classification: (firstResult.identifier) with confidence: (firstResult.confidence)") } } }
This class initializes a Core ML model and sets up a Vision request to analyze an image. The classification results are then processed and can be utilized to provide feedback or trigger actions within the app.
To enhance user experience, ponder using asynchronous handling and providing visual feedback while the image is being processed. The beauty of using pre-trained models is that they come with exceptionally optimized parameters, allowing developers to achieve high accuracy with minimal effort.
Optimizing Model Performance with CoreML
Optimizing model performance in Core ML very important for ensuring that machine learning applications run smoothly and efficiently on Apple devices. One of the most effective ways to boost performance is through the use of quantization and pruning techniques, which help reduce the model size and improve inference speed without significantly sacrificing accuracy.
Quantization involves converting the model weights from floating-point precision (typically 32-bit) to a lower precision such as 16-bit or even 8-bit integers. This not only reduces the memory footprint of the model but also allows for faster computations on devices with limited processing power. Core ML provides built-in support for quantization, which can be easily enabled during the model conversion process.
Pruning refers to the technique of removing less significant weights or neurons from a model. This can lead to a more streamlined network that requires fewer resources to run. By employing pruning, developers can achieve a balance between performance and accuracy, allowing for a more responsive user experience in real-time applications.
To demonstrate how to optimize a model using Core ML, let’s ponder the following example. We’ll convert a model to use quantized weights. That’s done by using the Core ML tools framework and modifying the training script of your model. Here’s a simplified version of how you might implement quantization:
import CoreML // Load your trained model let model = try MLModel(contentsOf: modelURL) // Define a configuration with quantization let configuration = MLModelConfiguration() configuration.computeUnits = .all configuration.quantization = .int8 // Convert the model with quantization let quantizedModel = try MLModel(configuration: configuration) // Save the quantized model let quantizedModelURL = modelURL.deletingLastPathComponent().appendingPathComponent("QuantizedModel.mlmodel") try quantizedModel.write(to: quantizedModelURL)
In this example, we create a configuration for our model that specifies we want to use integer quantization. After loading the original model, we convert it and save the quantized version. This reduces the model’s size and speeds up inference, making it ideal for mobile applications.
Furthermore, profiling the model’s performance is imperative to identify bottlenecks. Core ML allows you to integrate performance monitoring tools that can help you analyze how long the model takes to make predictions and how much memory it consumes. By using Instruments in Xcode, you can visualize and track the model’s performance metrics during runtime.
Another essential aspect of optimization is ensuring that your model is running on the appropriate hardware. Core ML supports running models on the CPU, GPU, or even the Neural Engine available in newer Apple devices. By configuring your model to utilize the Neural Engine, you can significantly enhance performance for supported tasks. This can be achieved with a simple change in the model’s configuration:
configuration.computeUnits = .neuralEngine
Integrating Vision Framework for Image Analysis
Integrating the Vision framework with Core ML opens a treasure trove of possibilities for image analysis and understanding. With the power of computer vision at your fingertips, you can build applications that not only recognize objects but also interpret scenes and extract meaningful information from images. The Vision framework simplifies the process of applying machine learning models to images, enabling developers to focus on the application layer rather than the intricacies of image processing.
To get started with integrating the Vision framework for image analysis, you’ll first want to ensure that you have a model that can perform the desired tasks, such as object detection or image segmentation. Once you have your model ready, you can easily integrate it using Vision.
Ponder the task of detecting objects in an image using a custom Core ML model. The process involves creating a Vision request that uses your model for inference. Here’s how you can implement this in Swift:
import CoreML import Vision import UIKit class ObjectDetector { private var model: VNCoreMLModel init() { guard let mlModel = try? YourCustomModel(configuration: MLModelConfiguration()) else { fatalError("Failed to load the model") } model = try! VNCoreMLModel(for: mlModel.model) } func detectObjects(in image: UIImage) { guard let ciImage = CIImage(image: image) else { fatalError("Failed to convert UIImage to CIImage") } let request = VNCoreMLRequest(model: model) { request, error in guard let results = request.results as? [VNRecognizedObjectObservation] else { fatalError("Unexpected results") } self.handleDetectionResults(results) } let handler = VNImageRequestHandler(ciImage: ciImage, options: [:]) do { try handler.perform([request]) } catch { print("Failed to perform detection: (error.localizedDescription)") } } private func handleDetectionResults(_ results: [VNRecognizedObjectObservation]) { for observation in results { let boundingBox = observation.boundingBox let confidence = observation.confidence let label = observation.labels.first?.identifier ?? "Unknown" print("Detected object: (label) with confidence: (confidence), bounding box: (boundingBox)") } } }
In this code snippet, we establish a new class called ObjectDetector
that initializes a Vision model for object detection. The detectObjects(in:)
method takes a UIImage, converts it to CIImage, and then creates a VNCoreMLRequest
to process the image. The results are handled in the handleDetectionResults(_:)
method, where you can access each detected object’s bounding box, confidence level, and label.
One crucial aspect to consider is the visualization of results. To provide immediate feedback to users, you can overlay bounding boxes and labels on the original image, enhancing the interactive experience. This can be done using UIView to draw rectangles and text based on the bounding box coordinates returned from the model.
The Vision framework also allows for advanced image analysis tasks such as face detection, text recognition, and feature point detection, all of which can be seamlessly combined with your Core ML models. By using the Vision framework, you gain access to a set of robust tools that can enhance your applications significantly without delving deep into the complexities of image processing algorithms.
Custom Model Training with Create ML
import CoreML import CreateML import Foundation class CustomModelTrainer { func trainModel() { // Load your training data let dataURL = URL(fileURLWithPath: "/path/to/your/data.json") let trainingData = try MLDataTable(contentsOf: dataURL) // Specify the model parameters let modelParameters = MLModelParameters() modelParameters.epochs = 50 modelParameters.batchSize = 32 modelParameters.learningRate = 0.01 // Train the model let model = try MLImageClassifier(trainingData: trainingData, parameters: modelParameters) // Save the trained model let modelURL = URL(fileURLWithPath: "/path/to/save/YourCustomModel.mlmodel") try model.write(to: modelURL) print("Model trained and saved successfully!") } }
Custom model training with Create ML is an empowering process that allows developers to tailor machine learning models specifically for their applications. Using Create ML simplifies the typically complex and resource-intensive aspects of model training, enabling even those with limited machine learning expertise to build robust models. Importantly, Create ML is designed to work seamlessly with Swift, making it an ideal choice for iOS and macOS developers.
To embark on custom model training, you begin by preparing your dataset. Depending on the task at hand—be it image classification, text analysis, or other applications—your data needs to be structured in a way that Create ML can interpret. For instance, when training an image classifier, you would typically organize your images in folders named after their corresponding labels.
Once you have your training data prepared, you can utilize the Create ML framework to define your model. The example above illustrates how to set up a custom image classifier. It starts by loading the training data from a specified URL, which can be in formats such as JSON, CSV, or even directly from image files.
Next, we create an instance of the MLImageClassifier
class, passing in the training data and defining model parameters like epochs
, batchSize
, and learningRate
. The epochs
parameter dictates how many times the model will see the entire dataset during training, while the batchSize
specifies how many samples will be processed before the model’s internal parameters are updated. The learningRate
is key in determining how quickly the model adjusts its weights during training, influencing both convergence speed and final model accuracy.
After executing the training process, the model can be saved to a file, making it ready for integration with your Core ML applications. The resulting model can then be utilized in a similar manner to pre-trained models, providing you the flexibility to customize the behavior and accuracy of the machine learning capabilities in your app.
One of the standout features of Create ML is its interactive playground environment, which allows you to visualize and iteratively improve your model’s performance. You can assess model accuracy, confusion matrices, and other metrics to understand how well your model performs across different classes in your dataset. This feedback loop is invaluable for refining your model and ensuring it meets the needs of your application.